- All Management Learning Resources
- Statistics for management
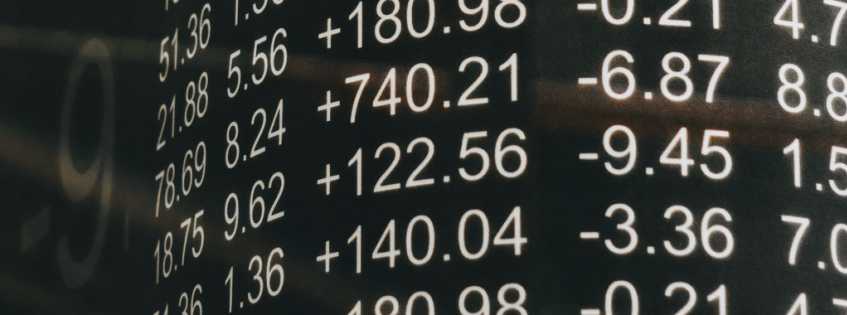
Why should you care about statistics for management?
To be an effective and analytical consumer of the literature on evidence-based management strategies, some familiarity and comfort with statistics is necessary. Statistical tests allow a researcher to test hypotheses and draw meaningful, logical conclusions from the data they have available. A rich panoply of statistical approaches exist, each tailored to specific types of data, study designs, and types of hypotheses. In this CQ Dossier you will learn the basics of statistics for management.
Contents
- Why should you care about statistics for management?
- Understanding science: Introduction to statistics for management
- T-Tests: Comparing averages between two groups
- Correlation: Measuring the extent to which two variables are associated with one another
- Regression: Describing the relationships between observed, measured variables
- ANOVA: Comparing means between groups
- P-Values: Measuring a statistical test’s significance
- Effect size measures: Estimating the magnitude and reproducibility of a study’s findings
- Confidence intervals: Estimating where the population’s true average score resides
- Key recommendations for professionals
- References and further reading
Understanding science: Introduction to statistics for management
While it is not necessary for a non-researcher to be a master at the computations underlying these statistics, some conceptual understanding is required. It’s immensely beneficial to grasp the basics of
- when a statistic is used,
- what kind of data each statistic can be used to analyze, and
- how statistics results are interpreted.
Below, these basic principles are described for some of the most commonly-used statistical tests in the social sciences: T-tests, correlations, regression, and ANOVA. Significance levels for each of these statistics are also described.
T-Tests: Comparing averages between two groups
When are t-tests used?
Simply put, t-tests compare averages between two groups. To compute a t-test, a researcher needs only the mean (arithmetic average) and standard deviation for each group being compared. The two groups being compared can be completely distinct experimental conditions, such as a treatment group and a control group, demographic categories (such as men and women), or even pre-test and post-test scores in a long-term study (longitudinal research design).
If the two groups being compared by the t-test are completely distinct, separate individuals, an independent-groups t-test is used; if the two averages being compared come from the same individuals at different points in time, a repeated-measures t-test is used. The formulas are slightly different to account for the distinct qualities of the samples, but both tests functionally compare averages.
How to interpret t-test results?
In a published journal article, reported t-test results usually take the following format:
University of Washington students taking statistics courses in Psychology had higher IQ scores (M = 121, SD = 14.2) than did those taking statistics courses in Statistics (M = 117, SD = 10.3), t(44) = 1.23, p = .09 (University of Washington, 2014).
In the above example, M and SD denote the means and standard deviations, respectively, and are reported for each of the two groups (Psychology students and Statistics students). The value in parentheses, 44, is a measure of the study’s degrees of freedom – essentially an adjusted record of the sample size. The value of the t-score, 1.23, indicates how far away the two group’s averages are from one another, in standard deviation units.
In this case, Psychology students’ scores were, on average, 1.23 standard deviations higher than Statistics’ students. A larger t-score, in absolute value, indicates a greater distance between the two groups. The p-value for this test is greater than .05, indicating that the difference is not statistically significant (see below for a more developed discussion of what a p-value symbolizes).
Correlation: Measuring the extent to which two variables are associated with one another
When is a correlation used?
Correlation measures the extent to which two variables are associated with one another. To use correlational analysis, both variables being examined must be measured and must exist along a continuum (in other words, they must be interval or ratio data, not categorical data).
If a researcher is concerned with whether two measurable factors are related to one another (for example, income and job satisfaction), correlation is frequently the correct approach.
How to interpret correlational results?
Correlations, represented by the letter r, are always between -1.0 and 1.0 in value; the absolute value (i.e., the distance from zero) of the correlation indicates the strength of the relationship between the two variables, with higher scores indicating stronger relationships.
The positivity (+) or negativity (-) of the correlation indicates the whether the variables are positively related or negatively related. A positive relationship indicates that as one variable increases, the other variable is expected to increase as well. A negative (or inverse) relationship indicates that as one variable increases in value, the other is expected to decrease.
Consider the following example of correlational results in a published article:
Hours spent studying and GPA (Great Point Average) were strongly positively correlated, r(123) = .61, p = .011. Hours spent playing video games and GPA were moderately negatively correlated, r(123) = .32, p = .041 (University of Washington, 2014).
In this example, r has a value of .61. This value is positive, indicating a positive relationship between hours spent studying and GPA. The value of .61 neither strong (between .8 – 1.0) nor weak (between 0.0 – 0.4); the relationship between these two variables is therefore moderate. The p-value for the correlation is less than .05, indicating that this observed relationship is statistically significant.
Regression: Describing the relationships between observed, measured variables
When is regression used?
Much like correlation, regression is used to describe the relationships between observed, measured variables. However, while a correlation can only examine the relationship between two variables at a time, regression can be used to build a complex model predicting an outcome variable using multiple predictor variables. Because regression can examine the combined predictive power of multiple variables at once, it can provide more accurate predictions of the outcome and describe how variables interact with one another.
How to interpret regression results?
Regression results are typically presented in a table, not in the text of a journal article. When examining these results, you can determine the strength and direction of the relationship between a predictor and the outcome variable by looking at the value for β (beta). Like a correlation coefficient, beta should be interpreted in terms of strength and direction – however, a beta score can be greater than 1.0.
ANOVA: Comparing means between groups
When is ANOVA used?
Analysis of variance (ANOVA) is used, much like a t-test, to compare means between groups of individuals. Whereas a t-test can only contrast two sets of scores, or compare two groups, ANOVA can be used to compare the averages of numerous groups, making it ideal for comparisons that involve numerous categories.
ANOVA can be used to, for example, compare the average incomes of employees in a variety of departments, or contrast the performance of multiple branches within an organization.
How to interpret ANOVA results?
ANOVA’s test statistic is call the F-ratio, and a higher F-ratio indicates a greater degree of difference between the multiple groups being compared. A value for F cannot be interpreted on its own; you must also examine whether the test was found to be statistically significant (see below for a discussion of p-values and statistical significance).
A significant ANOVA result only indicates that there is some notable difference between all the groups being compared; the test does not indicate on its own which groups are distinct from which other groups. Accordingly, a significant ANOVA must be followed with comparisons between individual pairings of groups, to locate where the meaningful differences are.
Here is an example write-up of ANOVA results:
An analysis of variance yielded a main effect for the diner’s race, F(1, 108) = 3.93, p < .05.
In this case, the computed value for F is 3.93, and the p-value was found to be less than .05, indicating statistical significance. These results indicate that the different racial groups examined in the study had significantly different average scores; follow-up tests would be required to determine which racial groups differed most from one another.
P-Values: Measuring a statistical test’s significance
A p-value, also known as a significance level or alpha level, is a measure of a statistical test’s significance. In most cases, a researcher aims to receive a significance level of .05 or lower; if a study’s observed p-value is below that threshold, observed results can be said to be statistically significant.
A p-value represents the computed odds of a Type I error, also known as a “false positive” -- in other words, the risk that a result that was found does not, in actuality, exist. A lower p-value, then, indicated a lower chance that a researcher’s observations were made in error.
Effect size measures: Estimating the magnitude and reproducibility of a study’s findings
In some areas of the social sciences, p-values have been supplanted in importance by effect size measures (Ferguson, 2009). These statistics provide the researcher with an estimate of the magnitude and reproducibility of a study’s findings. Unlike a p-value, which is applicable to nearly all statistics, there are specific effect size measures for each test statistic.
For example, in correlational analysis, the best way to measure effect size is to compute R-squared. As its name indicates, this effect size measure is computed by taking the correlation, r, and squaring it; the resulting number is interpreted as the percentage of variance explained by the study.
Every effect size measure is computed in a distinct way, but as a general rule, a higher score indicates that a more robust and large effect has been observed. Large effects are easier to locate in a study, require a smaller sample size to find, and are easier to reproduce.
Confidence intervals: Estimating where the population’s true average score resides
When are confidence intervals used?
Much like effect size measures, confidence intervals have risen in prominence in the social science literature in order to address the limitations of p-values (Du Prel, Hommel, Rohrig, & Blettner, 2009). A confidence interval is a range of scores, within which a population’s true score is estimated to reside.
Because most social scientific work is performed on samples that do not perfectly represent the population as a whole, means and data patterns located within a sample may not be entirely accurate or reproducible in the population itself.
How to interpret confidence intervals results?
Confidence intervals address this limitation by giving the researcher a range of possible scores; the population’s true mean is estimated to be within that range if scores, usually with approximately 95% accuracy.
In other words, a confidence interval is computed to estimate where the population’s true average score resides; the resulting range of scores can be expected to encapsulate the true population average in 95 out of 100 studies.
Confidence intervals can describe overall data trends, and indicate if meaningful differences exist between groups; they can also provide the researcher with information on the direction of that difference. As with p-values, confidence intervals can be used to draw conclusions about whether effects and differences are statistically significant.
An effect or group difference that is larger than the width of a confidence-interval is likely to be robust and reproducible, and can be considered to be statistically significant.
For example, if the treatment group and control group are 2.5 units away from one another, on average, and the confidence interval is only 1.2 units wide, the difference between the treatment and control groups can be concluded to be significant.
Key recommendations for professionals
- Statistical tests are selected by researchers on the basis of the type of data they have collected, and the nature of the research questions they are examining
- T-tests and ANOVAs are used to compare averages between distinct groups
- Correlation and regression are used to describe linear relationships between two measured variables
- A p-value is an estimate of a study’s “false positive” rate; a lower value (below .05) indicates greater significance
- Effect size measures and confidence intervals are becoming increasingly common in research reports, and indicate the robustness and reproducibility of a study’s observed effects
Management skills newsletter
Join our monthly newsletter to receive management tips, tricks and insights directly into your inbox!
References and further reading
“Choosing the correct statistical test in SAS, STAT, SPSS, and R.” (2017). UCLA Institute for Digital Research and Education. Retrieved from: https://stats.idre.ucla.edu/other/mult-pkg/whatstat/(link is external)
“Reporting Results of Common Statistical Tests in APA Format” (2014). University of Washington Psychology Writing Center. Retrieved from: https://depts.washington.edu/psych/files/writing_center/stats.pdf(link is external)
Du Prel, J.-B., Hommel, G., Röhrig, B., & Blettner, M. (2009). Confidence Interval or P-Value?: Part 4 of a Series on Evaluation of Scientific Publications. Deutsches Ärzteblatt International, 106(19), 335–339. http://doi.org/10.3238/arztebl.2009.0335(link is external)
Ferguson, C. J. (2009). An effect size primer: A guide for clinicians and researchers. Professional Psychology: Research and Practice, 40(5), 532.
Ramos-Álvarez, M. M., Moreno-Fernández, M. M., Valdés-Conroy, B., & Catena, A. (2008). Criteria of the peer review process for publication of experimental and quasi-experimental research in Psychology: A guide for creating research papers. International Journal of Clinical and Health Psychology, 8(3).
Sun, S., Pan, W., & Wang, L. L. (2010). A comprehensive review of effect size reporting and interpreting practices in academic journals in education and psychology. Journal of Educational Psychology, 102(4), 989.
Wilkinson, L. (1999). Statistical methods in psychology journals: Guidelines and explanations. American Psychologist, 54(8), 594.
About the Author
